Sampling inequalities affect generalization of neuroimaging-based ... - BMC Medicine
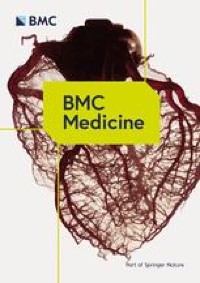
Jordan MI, Mitchell TM. Machine learning: trends, perspectives, and prospects. Science. 2015;349(6245):255–60.
Eyre HA, Singh AB, Reynolds C 3rd. Tech giants enter mental health. World Psychiatry. 2016;15(1):21–2.
Walter M, Alizadeh S, Jamalabadi H, Lueken U, Dannlowski U, Walter H, Olbrich S, Colic L, Kambeitz J, Koutsouleris N, et al. Translational machine learning for psychiatric neuroimaging. Prog Neuropsychopharmacol Biol Psychiatry. 2019;91:113–21.
Rutherford S. The promise of machine learning for psychiatry. Biol Psychiatry. 2020;88(11):e53–5.
Sui J, Jiang R, Bustillo J, Calhoun V. Neuroimaging-based individualized prediction of cognition and behavior for mental disorders and health: methods and promises. Biol Psychiatry. 2020;88(11):818–28.
Bzdok D, Varoquaux G, Steyerberg EW. Prediction, not association, paves the road to precision medicine. JAMA Psychiat. 2021;78(2):127–8.
Vayena E, Blasimme A. A systemic approach to the oversight of machine learning clinical translation. Am J Bioeth. 2022;22(5):23–5.
Poldrack RA, Huckins G, Varoquaux G. Establishment of best practices for evidence for prediction: a review. JAMA Psychiat. 2020;77(5):534–40.
Nielsen AN, Barch DM, Petersen SE, Schlaggar BL, Greene DJ. Machine learning with neuroimaging: evaluating its applications in psychiatry. Biol Psychiatry Cogn Neurosci Neuroimaging. 2020;5(8):791–8.
Varoquaux G, Raamana PR, Engemann DA, Hoyos-Idrobo A, Schwartz Y, Thirion B. Assessing and tuning brain decoders: cross-validation, caveats, and guidelines. Neuroimage. 2017;145(Pt B):166–79.
Dwyer DB, Falkai P, Koutsouleris N. Machine learning approaches for clinical psychology and psychiatry. Annu Rev Clin Psychol. 2018;14:91–118.
Mihalik A, Ferreira FS, Moutoussis M, Ziegler G, Adams RA, Rosa MJ, Prabhu G, de Oliveira L, Pereira M, Bullmore ET, et al. Multiple holdouts with stability: improving the generalizability of machine learning analyses of brain-behavior relationships. Biol Psychiatry. 2020;87(4):368–76.
Meehan AJ, Lewis SJ, Fazel S, Fusar-Poli P, Steyerberg EW, Stahl D, Danese A. Clinical prediction models in psychiatry: a systematic review of two decades of progress and challenges. Mol Psychiatry. 2022;27(6):2700–8.
Yuan J, Ran X, Liu K, Yao C, Yao Y, Wu H, Liu Q. Machine learning applications on neuroimaging for diagnosis and prognosis of epilepsy: A review. J Neurosci Methods. 2022;368:109441.
Davatzikos C. Machine learning in neuroimaging: Progress and challenges. Neuroimage. 2019;197:652–6.
Shrout PE, Rodgers JL. Psychology, science, and knowledge construction: broadening perspectives from the replication crisis. Annu Rev Psychol. 2018;69:487–510.
Maxwell SE, Lau MY, Howard GS. Is psychology suffering from a replication crisis? What does "failure to replicate" really mean? Am Psychol. 2015;70(6):487–98.
Henrich J, Heine SJ, Norenzayan A. Most people are not WEIRD. Nature. 2010;466(7302):29.
Muthukrishna M, Bell AV, Henrich J, Curtin CM, Gedranovich A, McInerney J, Thue B. Beyond Western, Educated, Industrial, Rich, and Democratic (WEIRD) psychology: measuring and mapping scales of cultural and psychological distance. Psychol Sci. 2020;31(6):678–701.
Rad MS, Martingano AJ, Ginges J. Toward a psychology of Homo sapiens: making psychological science more representative of the human population. Proc Natl Acad Sci U S A. 2018;115(45):11401–5.
Arnett JJ. The neglected 95%: why American psychology needs to become less American. Am Psychol. 2008;63(7):602–14.
Chen Z, Liu X, Yang Q, Wang YJ, Miao K, Gong Z, Yu Y, Leonov A, Liu C, Feng Z, et al. Evaluation of risk of bias in neuroimaging-based artificial intelligence models for psychiatric diagnosis: a systematic review. JAMA Netw Open. 2023;6(3):e231671.
Cearns M, Hahn T, Baune BT. Recommendations and future directions for supervised machine learning in psychiatry. Transl Psychiatry. 2019;9(1):271.
Tiwari P, Verma R. The pursuit of generalizability to enable clinical translation of radiomics. Radiol Artif Intell. 2021;3(1):e200227.
Wolfers T, Doan NT, Kaufmann T, Alnæs D, Moberget T, Agartz I, Buitelaar JK, Ueland T, Melle I, Franke B, et al. Mapping the heterogeneous phenotype of schizophrenia and bipolar disorder using normative models. JAMA Psychiat. 2018;75(11):1146–55.
Schultebraucks K, Choi KW, Galatzer-Levy IR, Bonanno GA. Discriminating heterogeneous trajectories of resilience and depression after major life stressors using polygenic scores. JAMA Psychiat. 2021;78(7):744–52.
Lee HB, Lyketsos CG. Depression in Alzheimer's disease: heterogeneity and related issues. Biol Psychiatry. 2003;54(3):353–62.
Arguello PA, Gogos JA. Genetic and cognitive windows into circuit mechanisms of psychiatric disease. Trends Neurosci. 2012;35(1):3–13.
Ying X. An overview of overfitting and its solutions. J Phys: Conf Ser. 2019;1168(2):022022.
Peng Y, Nagata MH. An empirical overview of nonlinearity and overfitting in machine learning using COVID-19 data. Chaos, Solitons Fractals. 2020;139:110055.
Andaur Navarro CL, Damen JAA, Takada T, Nijman SWJ, Dhiman P, Ma J, Collins GS, Bajpai R, Riley RD, Moons KGM, et al. Risk of bias in studies on prediction models developed using supervised machine learning techniques: systematic review. BMJ. 2021;375:n2281.
Hosseini M, Powell M, Collins J, Callahan-Flintoft C, Jones W, Bowman H, Wyble B. I tried a bunch of things: the dangers of unexpected overfitting in classification of brain data. Neurosci Biobehav Rev. 2020;119:456–67.
Varoquaux G, Cheplygina V. Machine learning for medical imaging: methodological failures and recommendations for the future. NPJ Digit Med. 2022;5(1):48.
Varoquaux G. Cross-validation failure: small sample sizes lead to large error bars. Neuroimage. 2018;180(Pt A):68–77.
Chen J, Patil KR, Yeo BTT, Eickhoff SB. Leveraging machine learning for gaining neurobiological and nosological insights in psychiatric research. Biol Psychiatry. 2023;93(1):18–28.
Page MJ, McKenzie JE, Bossuyt PM, Boutron I, Hoffmann TC, Mulrow CD, Shamseer L, Tetzlaff JM, Akl EA, Brennan SE, et al. The PRISMA 2020 statement: an updated guideline for reporting systematic reviews. BMJ. 2021;372:n71.
Moons KG, de Groot JA, Bouwmeester W, Vergouwe Y, Mallett S, Altman DG, Reitsma JB, Collins GS. Critical appraisal and data extraction for systematic reviews of prediction modelling studies: the CHARMS checklist. PLoS Med. 2014;11(10):e1001744.
Collins GS, Reitsma JB, Altman DG, Moons KG. Transparent reporting of a multivariable prediction model for individual prognosis or diagnosis (TRIPOD): the TRIPOD statement. BMJ. 2015;350:g7594.
Nam CW. World Economic Outlook for 2020 and 2021. CESifo Forum. 2020;21(2):58-9. https://www.proquest.com/openview/2b714d1282ff098661c0d252c4db128b/1?cbl=43805&pq-origsite=gscholar&parentSessionId=7a4xwuy%2B60cPGopgOGEQ6SUez3gxXxwiOjjkxULCRuI%3D.
How does the world bank classify countries? https://papers.ssrn.com/sol3/papers.cfm?abstract_id=2741183.
Dagum C. A new approach to the decomposition of the Gini income inequality ratio. Empir Econ. 1997;22:515–31.
Hamed KH, Ramachandra Rao A. A modified Mann-Kendall trend test for autocorrelated data. J Hydrol. 1998;204(1):182–96.
Battle DE. Diagnostic and Statistical Manual of Mental Disorders (DSM). CoDAS. 2013;25(2):191–2.
Huang Y, Wang Y, Wang H, Liu Z, Yu X, Yan J, Yu Y, Kou C, Xu X, Lu J, et al. Prevalence of mental disorders in China: a cross-sectional epidemiological study. Lancet Psychiatry. 2019;6(3):211–24.
Ormel J, VonKorff M. Reducing common mental disorder prevalence in populations. JAMA Psychiat. 2021;78(4):359–60.
Flint C, Cearns M, Opel N, Redlich R, Mehler DMA, Emden D, Winter NR, Leenings R, Eickhoff SB, Kircher T, et al. Systematic misestimation of machine learning performance in neuroimaging studies of depression. Neuropsychopharmacology. 2021;46(8):1510–7.
Woo CW, Chang LJ, Lindquist MA, Wager TD. Building better biomarkers: brain models in translational neuroimaging. Nat Neurosci. 2017;20(3):365–77.
Collins GS, Dhiman P, Andaur Navarro CL, Ma J, Hooft L, Reitsma JB, Logullo P, Beam AL, Peng L, Van Calster B, et al. Protocol for development of a reporting guideline (TRIPOD-AI) and risk of bias tool (PROBAST-AI) for diagnostic and prognostic prediction model studies based on artificial intelligence. BMJ Open. 2021;11(7):e048008.
Poldrack RA, Baker CI, Durnez J, Gorgolewski KJ, Matthews PM, Munafò MR, Nichols TE, Poline JB, Vul E, Yarkoni T. Scanning the horizon: towards transparent and reproducible neuroimaging research. Nat Rev Neurosci. 2017;18(2):115–26.
Knoth IS, Lajnef T, Rigoulot S, Lacourse K, Vannasing P, Michaud JL, Jacquemont S, Major P, Jerbi K, Lippé S. Auditory repetition suppression alterations in relation to cognitive functioning in fragile X syndrome: a combined EEG and machine learning approach. J Neurodev Disord. 2018;10(1):4.
Pedersen M, Curwood EK, Archer JS, Abbott DF, Jackson GD. Brain regions with abnormal network properties in severe epilepsy of Lennox-Gastaut phenotype: multivariate analysis of task-free fMRI. Epilepsia. 2015;56(11):1767–73.
Wang Y, Yuan L, Shi J, Greve A, Ye J, Toga AW, Reiss AL, Thompson PM. Applying tensor-based morphometry to parametric surfaces can improve MRI-based disease diagnosis. Neuroimage. 2013;74:209–30.
Hoeft F, Walter E, Lightbody AA, Hazlett HC, Chang C, Piven J, Reiss AL. Neuroanatomical differences in toddler boys with fragile x syndrome and idiopathic autism. Arch Gen Psychiatry. 2011;68(3):295–305.
Parisot S, Ktena SI, Ferrante E, Lee M, Guerrero R, Glocker B, Rueckert D. Disease prediction using graph convolutional networks: application to autism spectrum disorder and Alzheimer's disease. Med Image Anal. 2018;48:117–30.
Matlis S, Boric K, Chu CJ, Kramer MA. Robust disruptions in electroencephalogram cortical oscillations and large-scale functional networks in autism. BMC Neurol. 2015;15:97.
Ingalhalikar M, Parker D, Bloy L, Roberts TP, Verma R. Diffusion based abnormality markers of pathology: toward learned diagnostic prediction of ASD. Neuroimage. 2011;57(3):918–27.
Shahamat H, Saniee Abadeh M. Brain MRI analysis using a deep learning based evolutionary approach. Neural Netw. 2020;126:218–34.
Bajestani GS, Behrooz M, Khani AG, Nouri-Baygi M, Mollaei A. Diagnosis of autism spectrum disorder based on complex network features. Comput Methods Programs Biomed. 2019;177:277–83.
Lanka P, Rangaprakash D, Dretsch MN, Katz JS, Denney TS Jr, Deshpande G. Supervised machine learning for diagnostic classification from large-scale neuroimaging datasets. Brain Imaging Behav. 2020;14(6):2378–416.
Zhang L, Wang XH, Li L. Diagnosing autism spectrum disorder using brain entropy: a fast entropy method. Comput Methods Programs Biomed. 2020;190:105240.
Rakić M, Cabezas M, Kushibar K, Oliver A, Lladó X. Improving the detection of autism spectrum disorder by combining structural and functional MRI information. NeuroImage Clin. 2020;25:102181.
Ahmadlou M, Adeli H, Adeli A. Fractality and a wavelet-chaos-neural network methodology for EEG-based diagnosis of autistic spectrum disorder. J Clin Neurophysiol. 2010;27(5):328–33.
Libero LE, DeRamus TP, Lahti AC, Deshpande G, Kana RK. Multimodal neuroimaging based classification of autism spectrum disorder using anatomical, neurochemical, and white matter correlates. Cortex. 2015;66:46–59.
Pham TH, Vicnesh J, Wei JKE, Oh SL, Arunkumar N, Abdulhay EW, Ciaccio EJ, Acharya UR. Autism Spectrum Disorder Diagnostic System Using HOS Bispectrum with EEG Signals. Int J Environ Res Public Health. 2020;17(3):971.
Graa O, Rekik I. Multi-view learning-based data proliferator for boosting classification using highly imbalanced classes. J Neurosci Methods. 2019;327:108344.
Ingalhalikar M, Smith AR, Bloy L, Gur R, Roberts TP, Verma R. Identifying sub-populations via unsupervised cluster analysis on multi-edge similarity graphs. Med Image Comput Comput Assist Interv. 2012;15(Pt 2):254–61.
Khosla M, Jamison K, Kuceyeski A, Sabuncu MR. Ensemble learning with 3D convolutional neural networks for functional connectome-based prediction. Neuroimage. 2019;199:651–62.
Li H, Parikh NA, He L. A novel transfer learning approach to enhance deep neural network classification of brain functional connectomes. Front Neurosci. 2018;12:491.
Sen B, Borle NC, Greiner R, Brown MRG. A general prediction model for the detection of ADHD and Autism using structural and functional MRI. PLoS One. 2018;13(4):e0194856.
Xu L, Hua Q, Yu J, Li J. Classification of autism spectrum disorder based on sample entropy of spontaneous functional near infra-red spectroscopy signal. Clin Neurophysiol. 2020;131(6):1365–74.
Ma X, Wang XH, Li L. Identifying individuals with autism spectrum disorder based on the principal components of whole-brain phase synchrony. Neurosci Lett. 2021;742:135519.
Rakhimberdina Z, Liu X, Murata AT. Population graph-based multi-model ensemble method for diagnosing autism spectrum disorder. Sensors (Basel). 2020;20(21):6001.
Tsiaras V, Simos PG, Rezaie R, Sheth BR, Garyfallidis E, Castillo EM, Papanicolaou AC. Extracting biomarkers of autism from MEG resting-state functional connectivity networks. Comput Biol Med. 2011;41(12):1166–77.
Wang H, Chen C, Fushing H. Extracting multiscale pattern information of fMRI based functional brain connectivity with application on classification of autism spectrum disorders. PLoS One. 2012;7(10):e45502.
Hu J, Cao L, Li T, Liao B, Dong S, Li P. Interpretable learning approaches in resting-state functional connectivity analysis: the case of autism spectrum disorder. Comput Math Methods Med. 2020;2020:1394830.
Jung M, Tu Y, Park J, Jorgenson K, Lang C, Song W, Kong J. Surface-based shared and distinct resting functional connectivity in attention-deficit hyperactivity disorder and autism spectrum disorder. Br J Psychiatry. 2019;214(6):339–44.
Heinsfeld AS, Franco AR, Craddock RC, Buchweitz A, Meneguzzi F. Identification of autism spectrum disorder using deep learning and the ABIDE dataset. NeuroImage Clinical. 2018;17:16–23.
Bhaumik R, Pradhan A, Das S, Bhaumik DK. Predicting autism spectrum disorder using domain-adaptive cross-site evaluation. Neuroinformatics. 2018;16(2):197–205.
Wang L, Wee CY, Tang X, Yap PT, Shen D. Multi-task feature selection via supervised canonical graph matching for diagnosis of autism spectrum disorder. Brain Imaging Behav. 2016;10(1):33–40.
Ecker C, Rocha-Rego V, Johnston P, Mourao-Miranda J, Marquand A, Daly EM, Brammer MJ, Murphy C, Murphy DG. Investigating the predictive value of whole-brain structural MR scans in autism: a pattern classification approach. Neuroimage. 2010;49(1):44–56.
Payabvash S, Palacios EM, Owen JP, Wang MB, Tavassoli T, Gerdes M, Brandes-Aitken A, Cuneo D, Marco EJ, Mukherjee P. White matter connectome edge density in children with autism spectrum disorders: potential imaging biomarkers using machine-learning models. Brain Connect. 2019;9(2):209–20.
Price T, Wee CY, Gao W, Shen D. Multiple-network classification of childhood autism using functional connectivity dynamics. Med Image Comput Comput Assist Interv. 2014;17(Pt 3):177–84.
Haweel R, Shalaby A, Mahmoud A, Seada N, Ghoniemy S, Ghazal M, Casanova MF, Barnes GN, El-Baz A. A robust DWT-CNN-based CAD system for early diagnosis of autism using task-based fMRI. Med Phys. 2021;48(5):2315–26.
Chen CP, Keown CL, Jahedi A, Nair A, Pflieger ME, Bailey BA, Müller RA. Diagnostic classification of intrinsic functional connectivity highlights somatosensory, default mode, and visual regions in autism. NeuroImage Clin. 2015;8:238–45.
Anderson JS, Nielsen JA, Froehlich AL, DuBray MB, Druzgal TJ, Cariello AN, Cooperrider JR, Zielinski BA, Ravichandran C, Fletcher PT, et al. Functional connectivity magnetic resonance imaging classification of autism. Brain. 2011;134(Pt 12):3742–54.
Uddin LQ, Supekar K, Lynch CJ, Khouzam A, Phillips J, Feinstein C, Ryali S, Menon V. Salience network-based classification and prediction of symptom severity in children with autism. JAMA Psychiat. 2013;70(8):869–79.
Nielsen JA, Zielinski BA, Fletcher PT, Alexander AL, Lange N, Bigler ED, Lainhart JE, Anderson JS. Multisite functional connectivity MRI classification of autism: ABIDE results. Front Hum Neurosci. 2013;7:599.
Jahedi A, Nasamran CA, Faires B, Fan J, Müller RA. Distributed intrinsic functional connectivity patterns predict diagnostic status in Large Autism Cohort. Brain Connect. 2017;7(8):515–25.
Retico A, Giuliano A, Tancredi R, Cosenza A, Apicella F, Narzisi A, Biagi L, Tosetti M, Muratori F, Calderoni S. The effect of gender on the neuroanatomy of children with autism spectrum disorders: a support vector machine case-control study. Mol Autism. 2016;7:5.
Calderoni S, Retico A, Biagi L, Tancredi R, Muratori F, Tosetti M. Female children with autism spectrum disorder: an insight from mass-univariate and pattern classification analyses. Neuroimage. 2012;59(2):1013–22.
Yamagata B, Itahashi T, Fujino J, Ohta H, Nakamura M, Kato N, Mimura M, Hashimoto RI, Aoki Y. Machine learning approach to identify a resting-state functional connectivity pattern serving as an endophenotype of autism spectrum disorder. Brain Imaging Behav. 2019;13(6):1689–98.
Gori I, Giuliano A, Muratori F, Saviozzi I, Oliva P, Tancredi R, Cosenza A, Tosetti M, Calderoni S, Retico A. Gray matter alterations in young children with autism spectrum disorders: comparing morphometry at the voxel and regional level. J Neuroimaging. 2015;25(6):866–74.
Comments
Post a Comment